Deep fake technology has taken the world by storm, reshaping how we perceive reality and fiction. Imagine a world where videos and images are so convincing that it's nearly impossible to tell what's real and what's fabricated. This isn't science fiction—it's happening right now. Deep fake is no longer just a buzzword; it’s a transformative force that’s reshaping industries, politics, and even our daily lives.
So, why should you care? Because understanding deep fake isn’t just about staying informed—it’s about staying ahead. In an era where misinformation spreads faster than truth, knowing how this technology works can empower you to make better decisions. Whether you're a tech enthusiast, a concerned citizen, or just someone curious about the future, this article dives deep into the world of deep fakes.
Here’s the deal: we’ll break down the technology behind deep fakes, explore its implications, and give you the tools to navigate this brave new world. By the end of this read, you’ll be equipped with the knowledge to separate fact from fiction—and maybe even impress your friends at the next dinner party.
Let’s jump in, shall we?
What Exactly Is Deep Fake Technology?
Deep fake refers to the use of artificial intelligence and machine learning to create realistic but fake videos, images, or audio. Think of it as digital sleight of hand, where algorithms can convincingly manipulate or generate content that looks authentic. The term "deep" comes from deep learning, a subset of AI that powers these creations, while "fake" speaks for itself.
At its core, deep fake technology uses neural networks to analyze vast amounts of data and replicate patterns. For instance, if you feed a system thousands of images of a person speaking, it can learn to generate new footage of that person saying something they never actually said. Sounds crazy, right? But that’s the power—and the danger—of deep fakes.
How Does Deep Fake Technology Work?
Behind every deep fake lies a complex process involving two key components: generative adversarial networks (GANs) and autoencoders. GANs consist of two neural networks: one generates fake content, while the other tries to detect it. Through constant competition, both networks improve, producing increasingly realistic results.
Autoencoders, on the other hand, compress and decompress data to extract essential features. Together, these tools enable deep fake creators to produce lifelike simulations that blur the line between reality and fabrication.
- GANs generate and refine fake content through competition.
- Autoencoders compress data to capture critical details.
- Both systems work together to create hyper-realistic outputs.
Why Should You Care About Deep Fakes?
The impact of deep fake technology extends far beyond entertainment. From political propaganda to identity theft, the potential misuse of this tech raises serious concerns. Imagine a world where a single deep fake video could sway public opinion, spark global unrest, or even influence elections. It’s not as far-fetched as it sounds.
But it’s not all doom and gloom. Deep fakes also offer exciting possibilities in fields like education, healthcare, and entertainment. Imagine personalized learning experiences or virtual simulations that enhance medical training. The key lies in responsible usage and regulation.
The Dark Side of Deep Fakes
One of the most alarming aspects of deep fakes is their ability to spread misinformation. A study by the Pew Research Center found that 59% of Americans believe fake news has caused significant confusion about basic facts. With deep fakes, this problem intensifies tenfold.
Moreover, deep fakes can be weaponized for malicious purposes, such as cyberbullying, revenge porn, or corporate sabotage. In 2020 alone, deep fake scams cost businesses over $25 million, according to a report by Symantec. These numbers highlight the urgent need for solutions.
The Evolution of Deep Fake Technology
Deep fake technology didn’t just appear overnight. Its roots trace back to the early days of computer vision and image processing. Over the years, advancements in AI and computing power have fueled its rapid development. Today, tools like Faceswap and DeepFaceLab make creating deep fakes accessible to virtually anyone with a computer.
But here’s the kicker: as the technology evolves, so do the challenges. Researchers are constantly working on detection methods to combat deep fakes, but it’s a never-ending arms race. The more sophisticated the fakes become, the harder they are to spot.
Key Milestones in Deep Fake Development
- 2014: Ian Goodfellow introduces GANs, laying the foundation for deep fakes.
- 2017: Reddit user "DeepFakes" popularizes the term with celebrity face swaps.
- 2020: Deep fake apps gain mainstream attention, sparking ethical debates.
Understanding the Science Behind Deep Fakes
For those who want to dive deeper, let’s break down the science behind deep fake technology. Neural networks, the backbone of AI, are designed to mimic the human brain’s ability to learn and adapt. By feeding these networks large datasets, they can recognize patterns and generate new content based on those patterns.
Data preprocessing plays a crucial role in this process. Raw data must be cleaned, labeled, and organized before being fed into the system. This step ensures the neural network learns from accurate and relevant information. Once trained, the network can produce outputs that mimic the input data, often with stunning accuracy.
Types of Deep Fakes
Not all deep fakes are created equal. Here’s a quick rundown of the different types:
- Face Swaps: Replacing one person’s face with another in videos or images.
- Lip Syncing: Making someone appear to say something they didn’t.
- Whole Body Manipulation: Altering entire body movements in videos.
- Audio Deep Fakes: Generating realistic but fake audio clips.
Real-World Applications of Deep Fakes
Despite its controversial reputation, deep fake technology has practical applications across various industries. In entertainment, filmmakers use deep fakes to de-age actors or bring deceased legends back to life. For example, Marvel Studios employed similar techniques to recreate young versions of Robert Downey Jr. and Samuel L. Jackson in "Avengers: Endgame."
In healthcare, deep fakes are being explored for speech therapy and patient simulations. By creating realistic avatars, therapists can provide personalized care without the need for physical presence. Similarly, educators are using deep fakes to create interactive learning materials that engage students in new ways.
Challenges and Opportunities
While the benefits are undeniable, the challenges remain significant. Balancing innovation with ethical considerations is no easy feat. Policymakers, technologists, and society as a whole must work together to establish guidelines and safeguards.
Some proposed solutions include watermarking deep fakes, developing advanced detection algorithms, and promoting digital literacy. These efforts aim to empower individuals to recognize and combat misinformation effectively.
How to Spot a Deep Fake
Identifying deep fakes isn’t always straightforward, but there are telltale signs to watch out for. Blurry edges around faces, unnatural blinking patterns, and inconsistent lighting are common red flags. Audio-video synchronization issues can also indicate tampering.
However, as deep fakes become more sophisticated, these indicators may disappear. That’s why researchers are developing AI-driven tools to detect even the most advanced fakes. Companies like Google and Microsoft are investing heavily in this area, hoping to stay one step ahead of bad actors.
Tools for Detecting Deep Fakes
- Forensic analysis software
- AI-powered detection platforms
- Blockchain-based verification systems
The Future of Deep Fake Technology
Looking ahead, the future of deep fake technology is both exciting and uncertain. As AI continues to advance, we can expect even more realistic and convincing creations. However, this progress brings with it increased risks and responsibilities.
Experts predict that deep fakes will play a significant role in shaping the digital landscape. From enhancing user experiences to redefining content creation, their impact will be felt across multiple domains. Yet, ensuring ethical use and protecting against misuse remains paramount.
Predictions for the Next Decade
- Deep fakes will become indistinguishable from reality.
- Regulations will tighten to address growing concerns.
- Public awareness and education will grow exponentially.
Conclusion: Navigating the Deep Fake Landscape
In conclusion, understanding deep fake technology is crucial in today’s information age. From its origins in AI research to its current applications and challenges, deep fakes represent a double-edged sword. While they offer incredible opportunities, they also pose significant threats.
To stay ahead, it’s essential to remain informed and vigilant. By educating yourself and others, you can help mitigate the risks associated with deep fakes. Share this article, engage in discussions, and advocate for responsible innovation. Together, we can shape a future where technology serves humanity, not the other way around.
Table of Contents
- What Exactly Is Deep Fake Technology?
- How Does Deep Fake Technology Work?
- Why Should You Care About Deep Fakes?
- The Dark Side of Deep Fakes
- The Evolution of Deep Fake Technology
- Understanding the Science Behind Deep Fakes
- Real-World Applications of Deep Fakes
- How to Spot a Deep Fake
- The Future of Deep Fake Technology
- Conclusion: Navigating the Deep Fake Landscape
Remember, knowledge is power. Stay curious, stay informed, and don’t forget to have fun exploring the world of deep fakes!
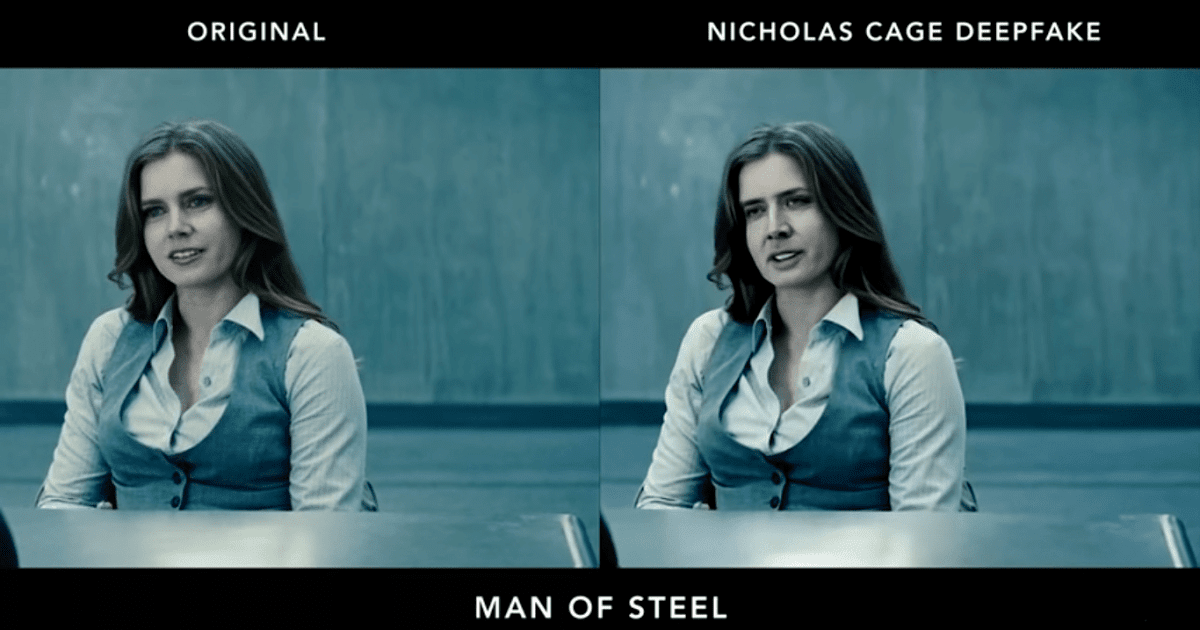

